Securing AI-based Security Systems
Key Points
- Fundamental weaknesses of AI include brittleness, embedded bias,
catastrophic forgetting and lack of explainability. - Although research is under way to address some of these issues, the
adoption of AI techniques and models in security systems exposes
potentially critical security systems to weaknesses/vulnerabilities such
as these. - Adversarial training is one strongly recommended approach to increase
the robustness (i.e. reduce the brittleness) of the AI model. In this
approach, the training dataset is extended to include adversarial
examples representative of potential attacks on the system. However, the
implementation of adversarial training is currently ad hoc. - Given the evidence of AI weaknesses, the omission of adversarial training
and similar hardening techniques for AI-based security systems is
unacceptable. Standardised testing and evaluation of AI-based security
systems is recommended. From a governance perspective, evidence of
adversarial robustness evaluation should be a minimum requirement for
the acceptance of an AI-based security system. - The production of strong adversarial samples does not account for
“black swan” events, i.e. random and unexpected events that have
an extreme impact. Given that security systems tend to be designed
to detect “old” or “known” types of attack, ways need to be found to
manage the occurrence of “new” attacks.
This is part of a series of publications for the Polymath Initiative.
Disclaimer: The views, information and opinions expressed in the written publications are the authors’ own and do not necessarily reflect those shared by the Geneva Centre for Security Policy or its employees. The GCSP is not responsible for and may not always verify the accuracy of the information contained in the written publications submitted by a writer.
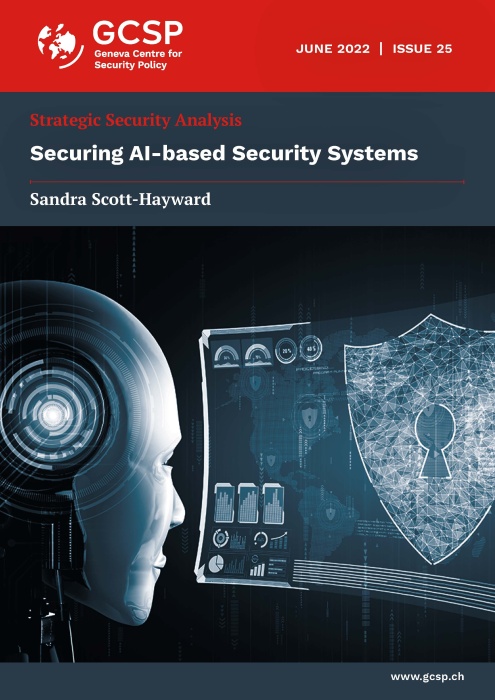
Dr Sandra Scott-Hayward is a GCSP Polymath Fellow and a Senior Lecturer (Associate Professor) with the School of Electronics, Electrical Engineering and Computer Science, and a Member of the Centre for Secure Information Technologies at Queen’s University Belfast (QUB). She is also the Director of the QUB Academic Centre of Excellence in Cyber Security Education.